NeurIPS 2023 Datasets and Benchmarks (Oral) Ethical Considerations for Responsible Data Curation Practical recommendations for responsibly curating human-centric computer vision datasets for fairness and robustness evaluations, addressing privacy and bias concerns
Abstract
Human-centric computer vision (HCCV) data curation practices often neglect privacy and bias concerns, leading to dataset retractions and unfair models. HCCV datasets constructed through nonconsensual web scraping lack crucial metadata for comprehensive fairness and robustness evaluations.
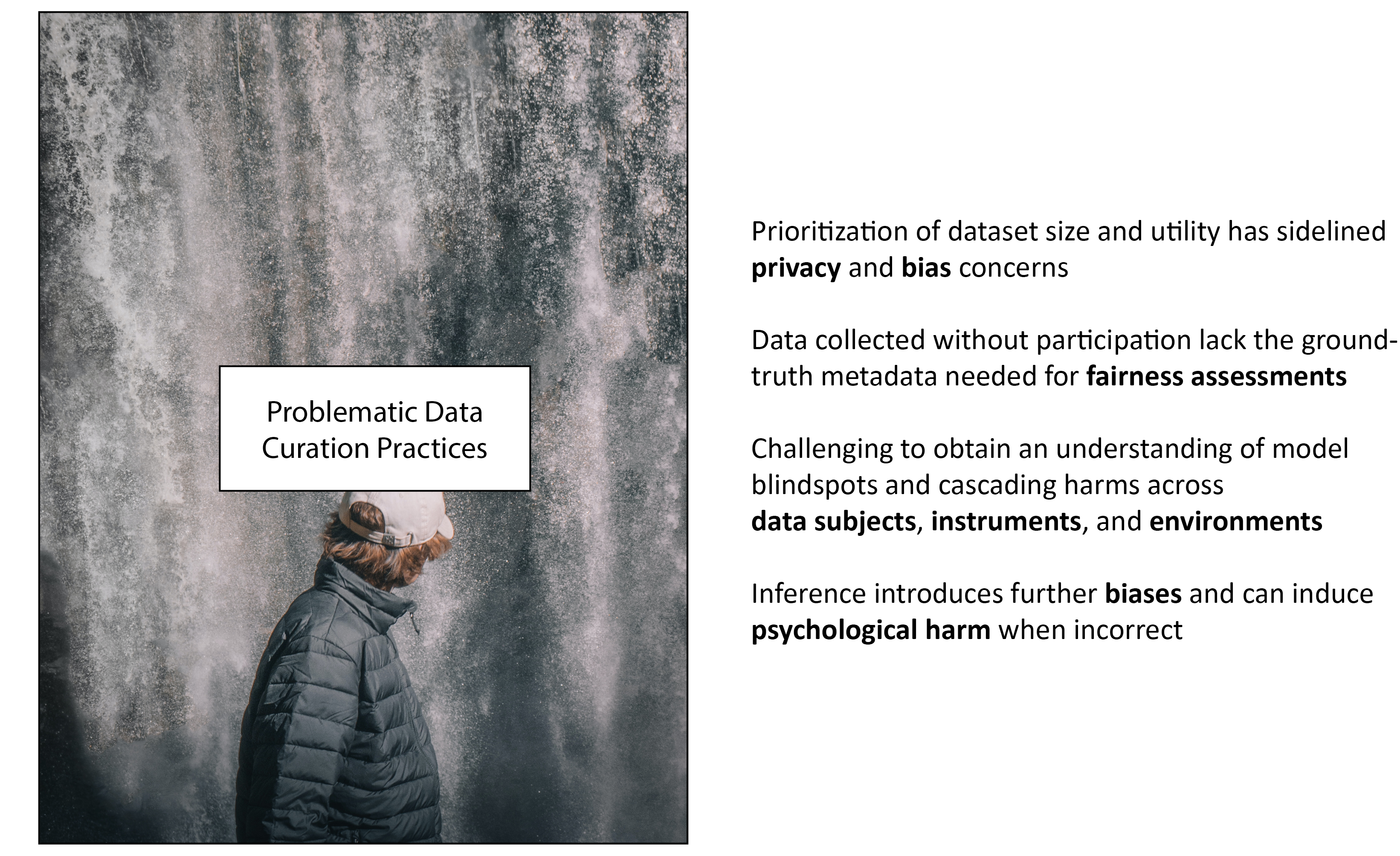
Current remedies are post hoc, lack persuasive justification for adoption, or fail to provide proper contextualization for appropriate application.
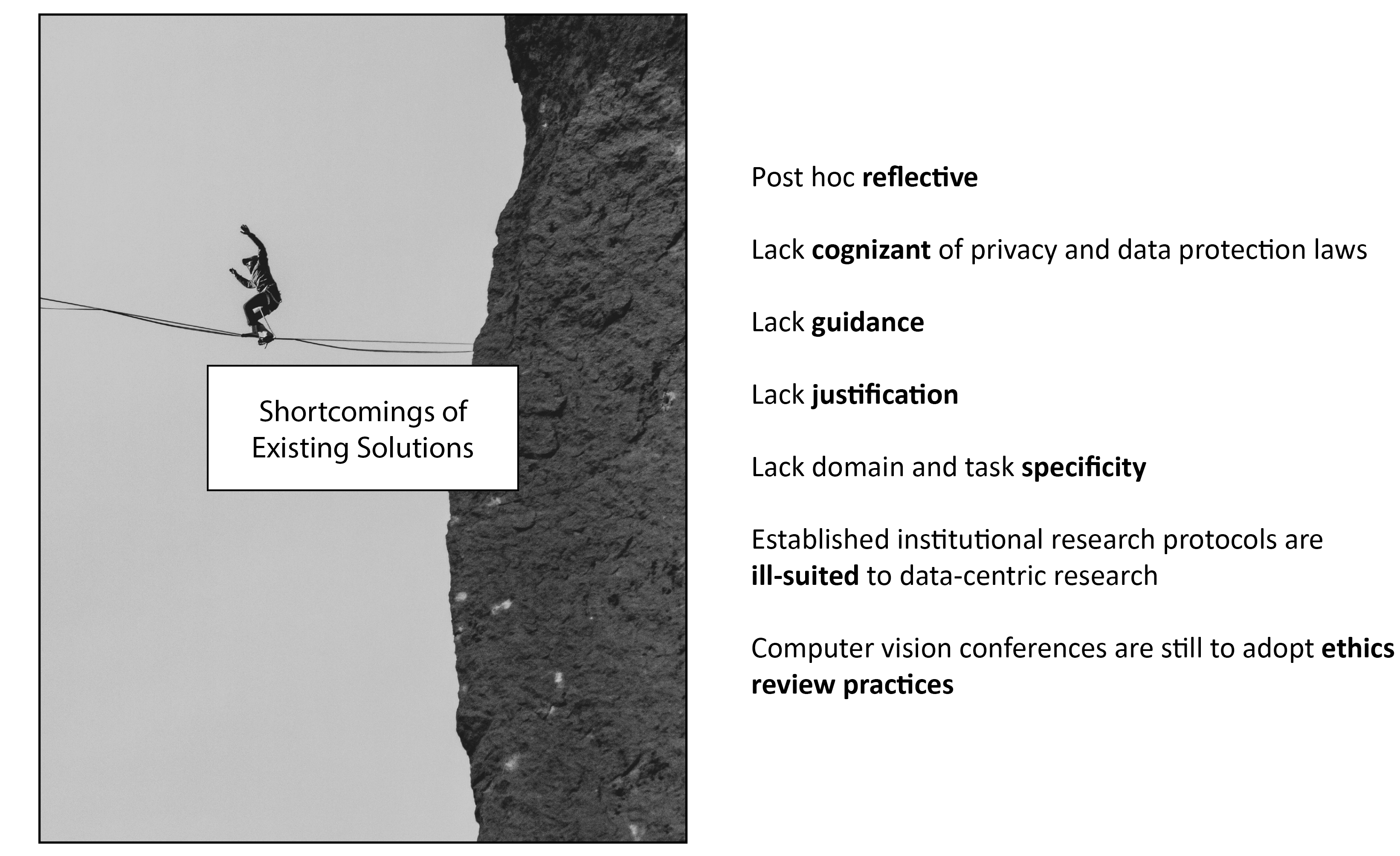
Our research focuses on proactive, domain-specific recommendations, covering purpose, privacy and consent, and diversity, for curating HCCV evaluation datasets, addressing privacy and bias concerns. We adopt an ante hoc reflective perspective, drawing from current practices, guidelines, dataset withdrawals, and audits, to inform our considerations and recommendations.
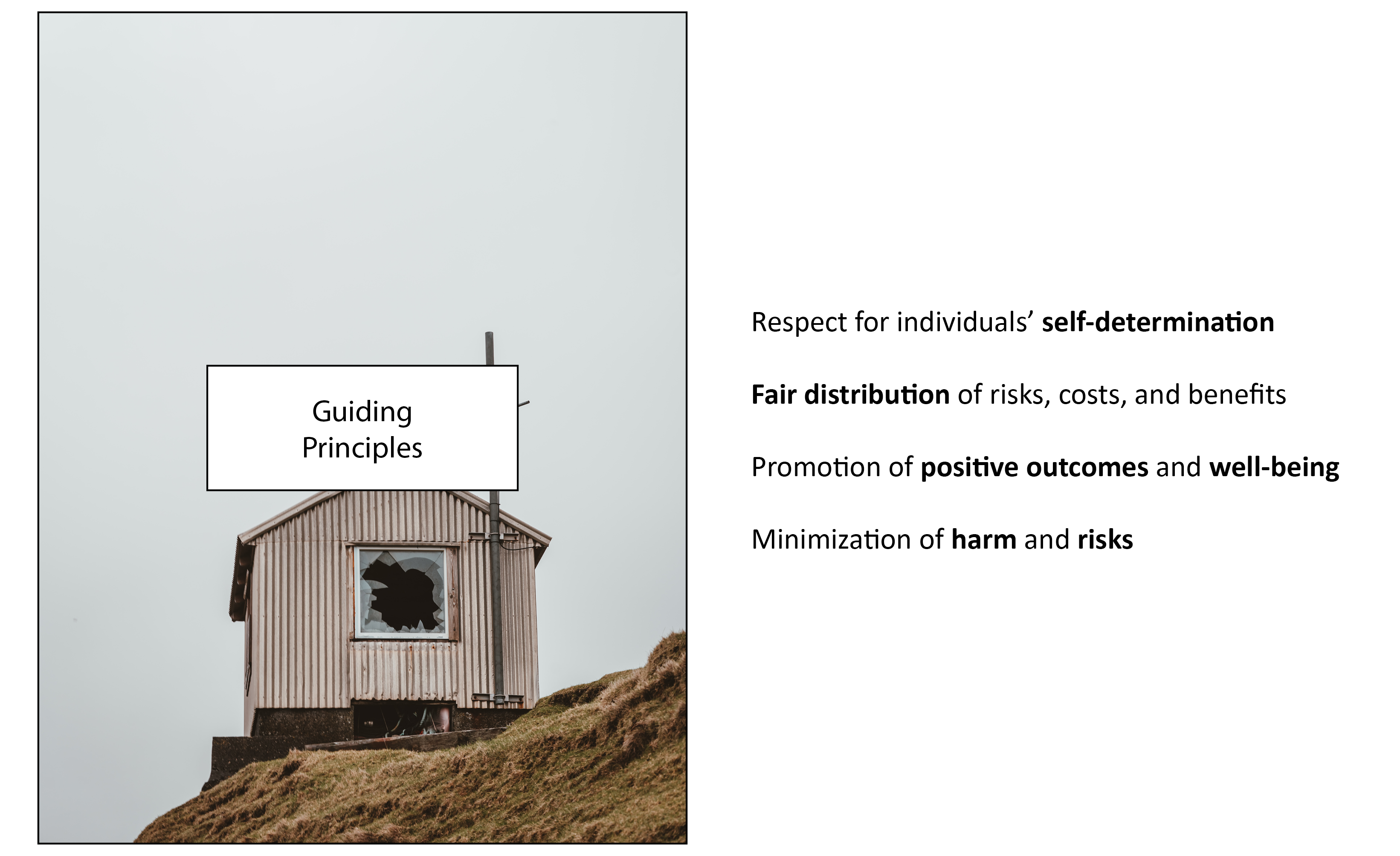
(To guide data curators towards more ethical yet resource-intensive curation, we also provide a checklist.)
It is important to make clear that our proposals are not intended for the evaluation of HCCV systems that detect, predict, or label sensitive or objectionable attributes such as race, gender, sexual orientation, or disability.
Considerations and Recommendations
Purpose
In ML, significant emphasis has been placed on the acquisition and utilization of "general-purpose" datasets1. Nevertheless, without a clearly defined task pre-data collection, it becomes challenging to effectively handle issues related to data composition, labeling, data collection methodologies, informed consent, and assessments related to data protection. We address conflicting dataset motivations and provide recommendations.
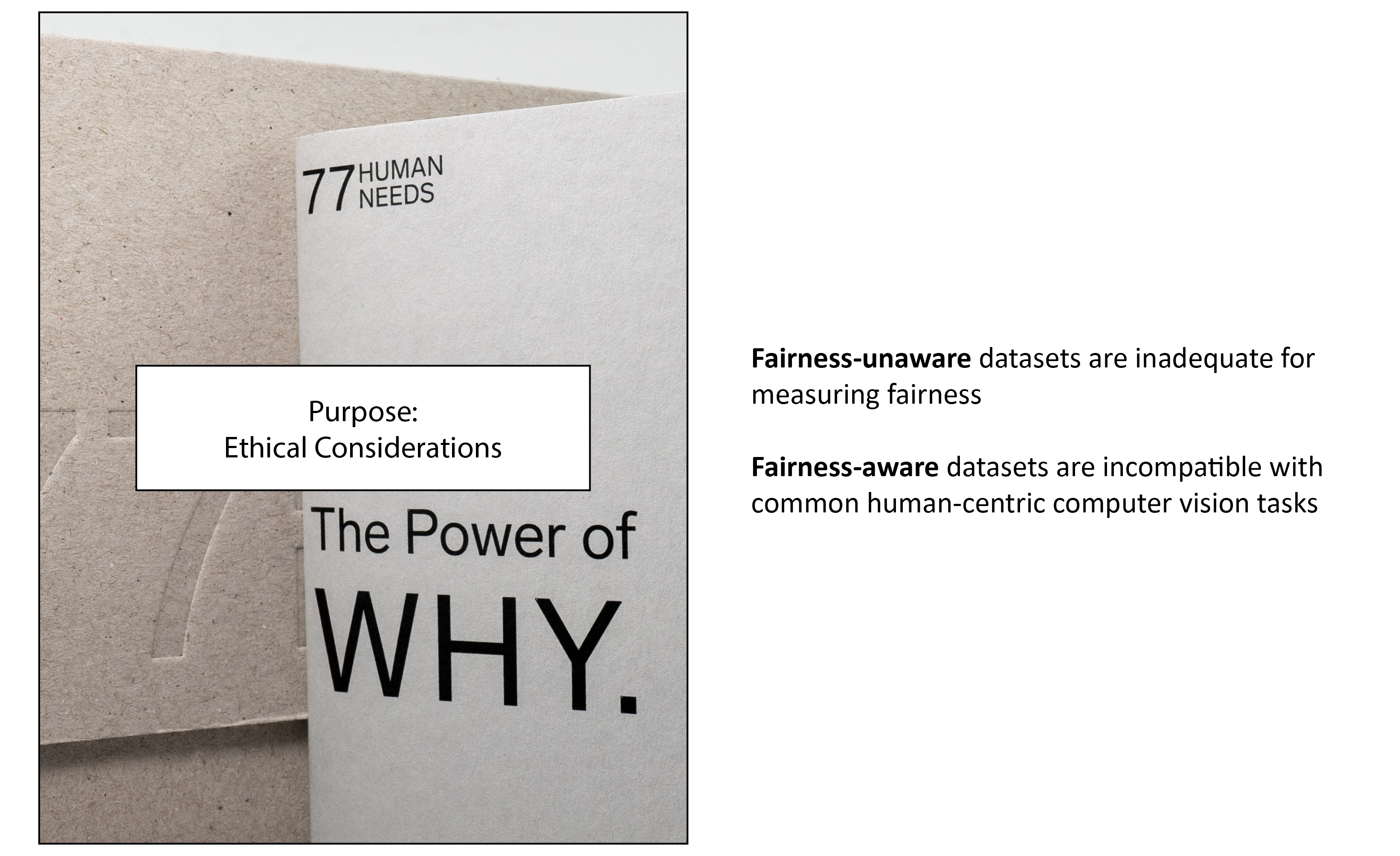
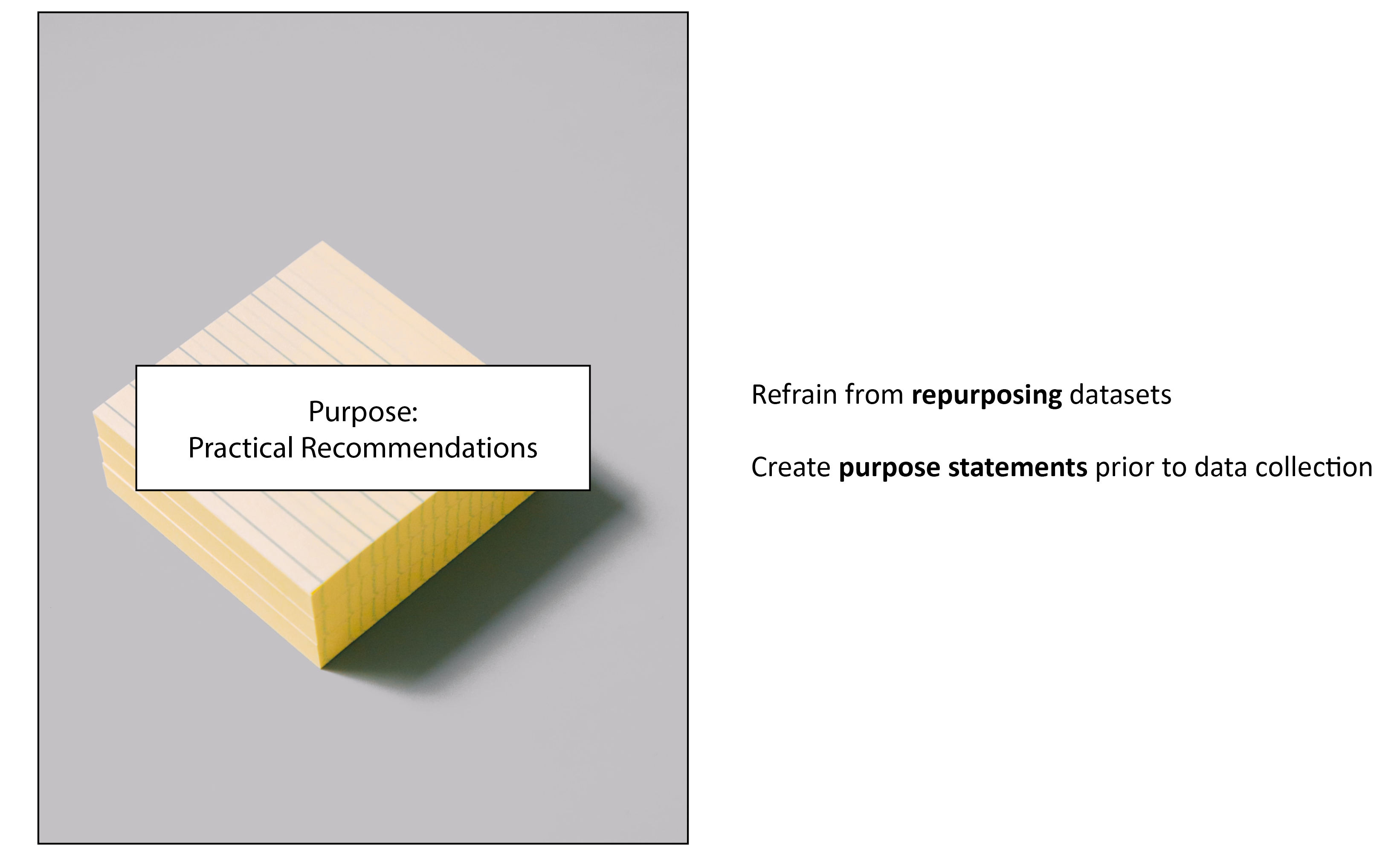
Consent and Privacy
Informed consent is crucial in research ethics involving humans2, 3, ensuring participant safety, protection, and research integrity4, 5. Shaping data collection practices in various fields(missing reference), informed consent consists of three elements: information (i.e., the participant should have sufficient knowledge about the study to make their decision), comprehension (i.e., the information about the study should be conveyed in an understandable manner), and voluntariness (i.e., consent must be given free of coercion or undue influence). While consent is not the only legal basis for data processing, it is globally preferred for its legitimacy and ability to foster trust5, 6. We address concerns related to consent and privacy, and provide recommendations.
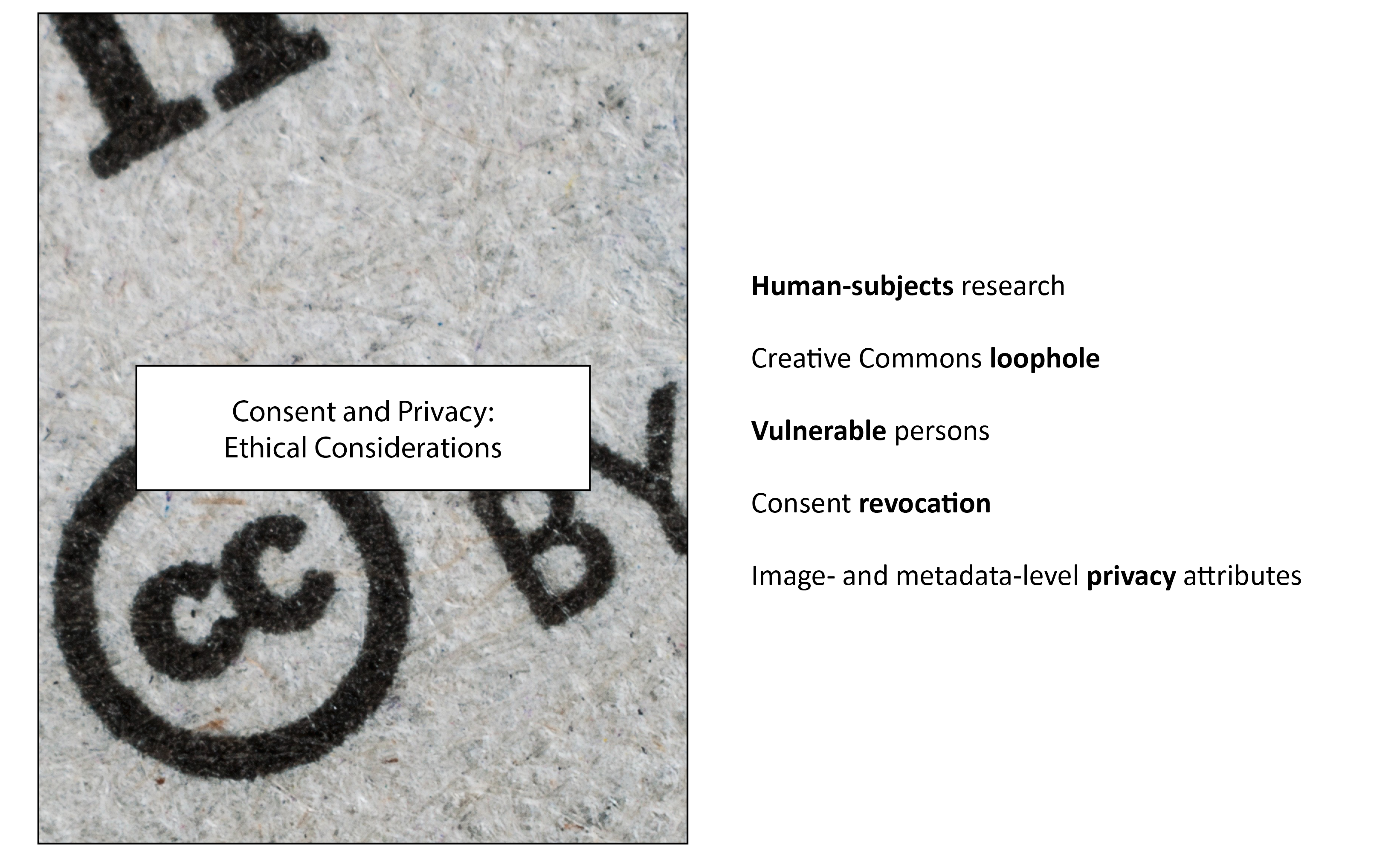
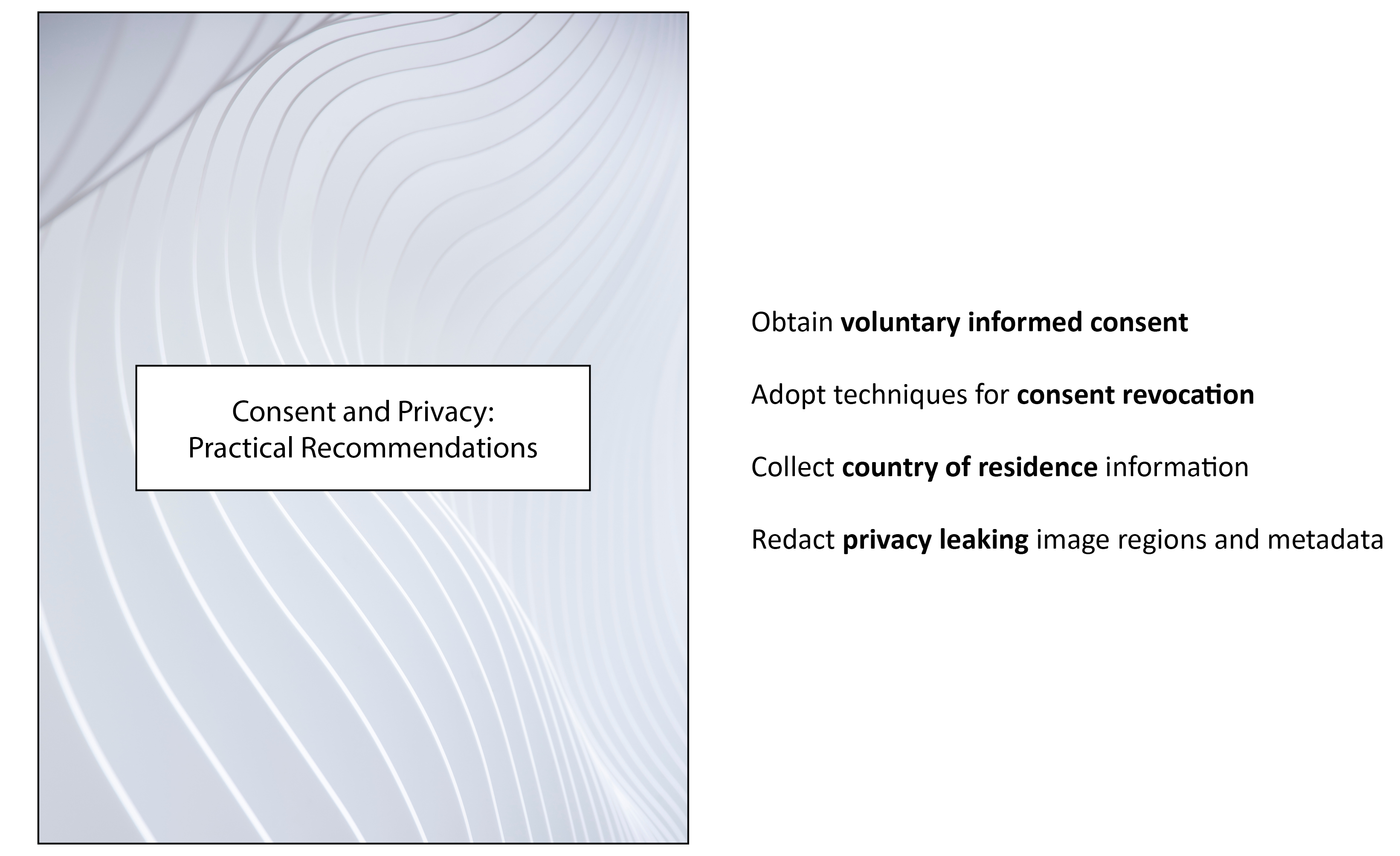
Diversity
HCCV dataset creators widely acknowledge the significance of dataset diversity7, 8, 9, 10, 11, 12, 13, 14, 15, 16, realism17, 18, 11, 16, 8, 19, and difficulty20, 8, 21, 22, 9, 23, 11, 12, 13, 15, 16, 19 to enhance fairness and robustness in real-world applications. Previous research has emphasized diversity across image subjects, environments, and instruments24, 25, 26, 27, but there are many ethical complexities involved in specifying diversity criteria28, 29, 30, 31. We examine taxonomy challenges and offer recommendations.
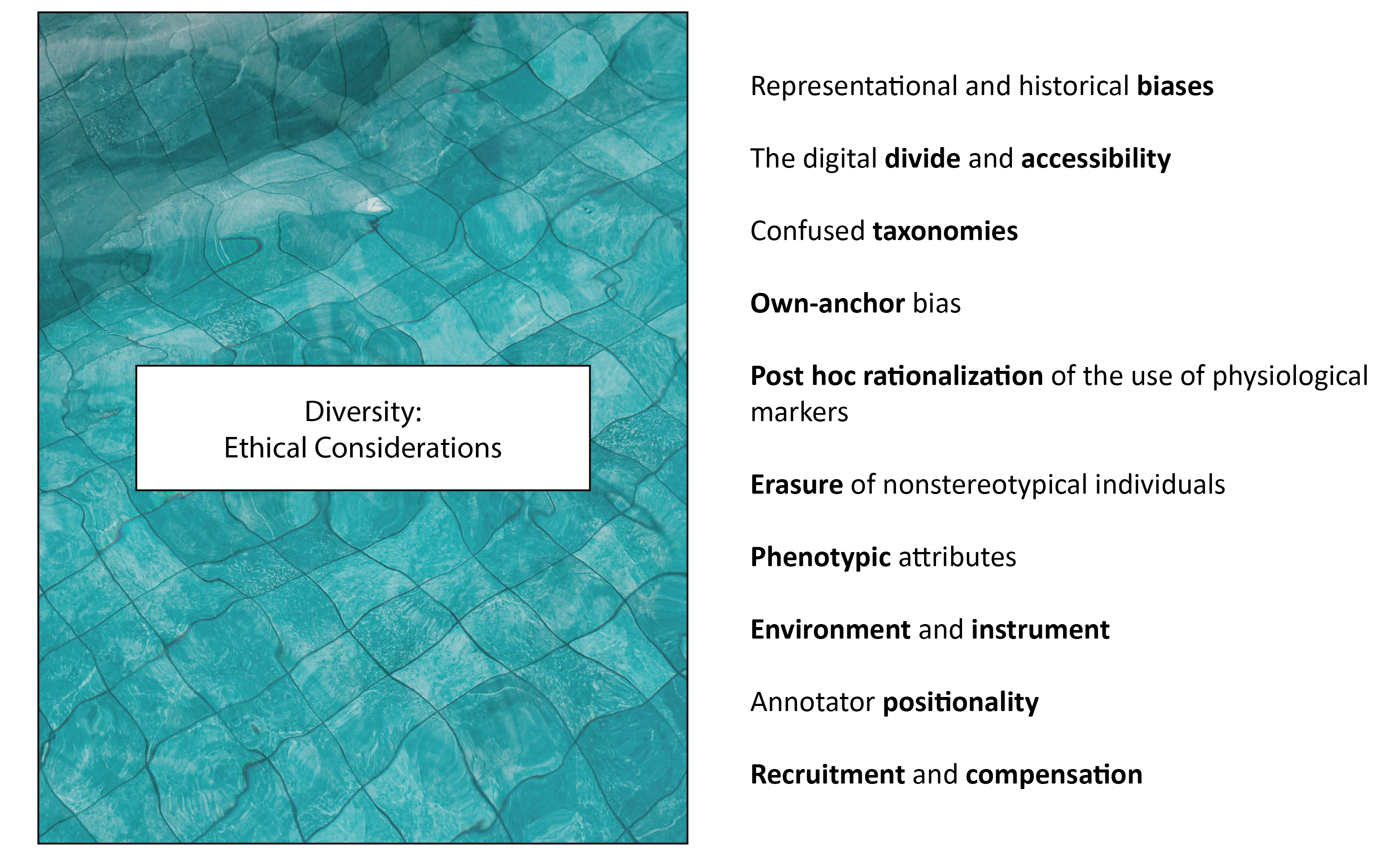
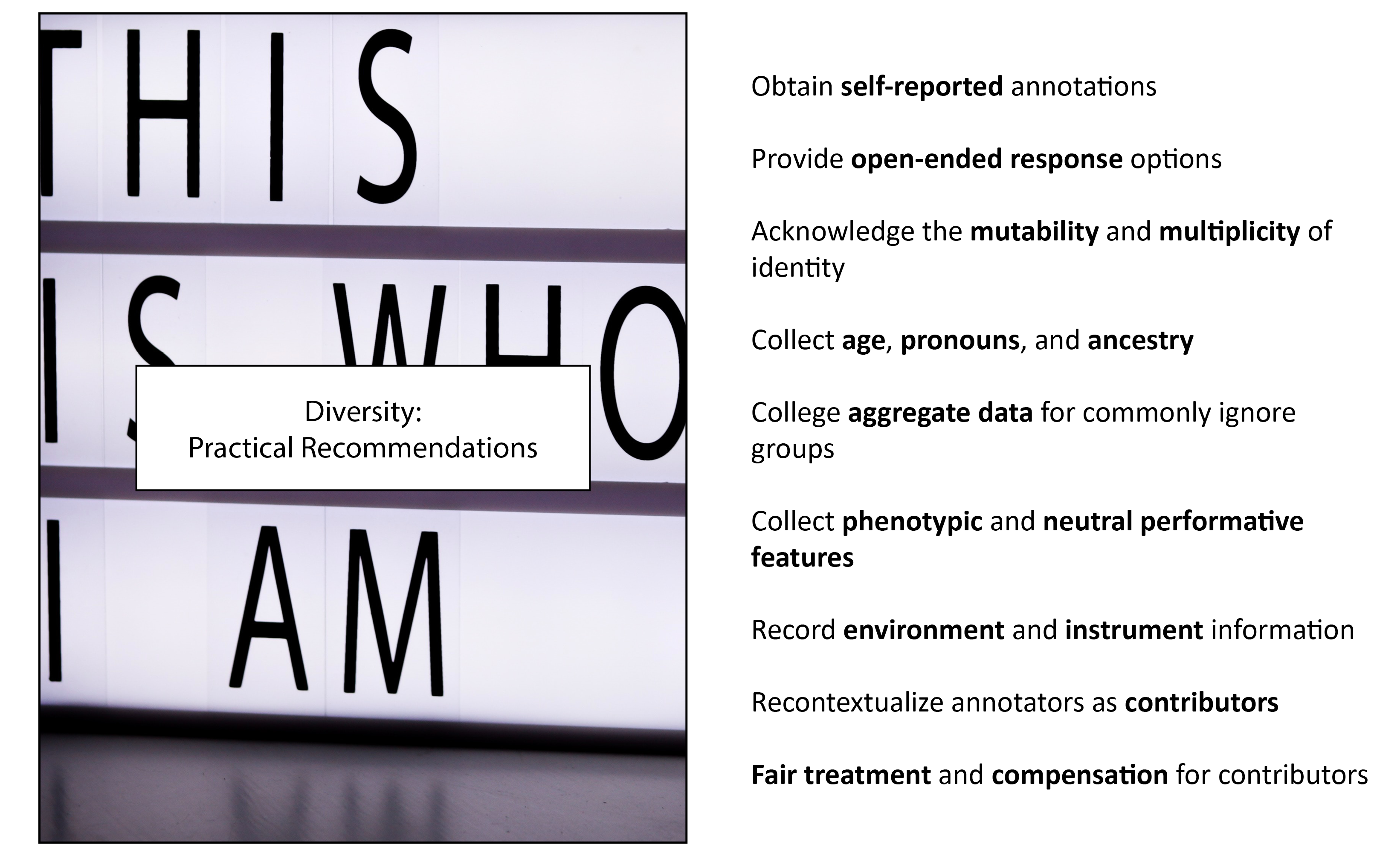
Concluding Remarks
Supplementary to established ethical review protocols, we have provided proactive, domain-specific recommendations for curating HCCV evaluation datasets for fairness and robustness evaluations. However, encouraging change in ethical practice could encounter resistance or slow adoption due to established norms32, inertia33, diffusion of responsibility34, and liability concerns29.
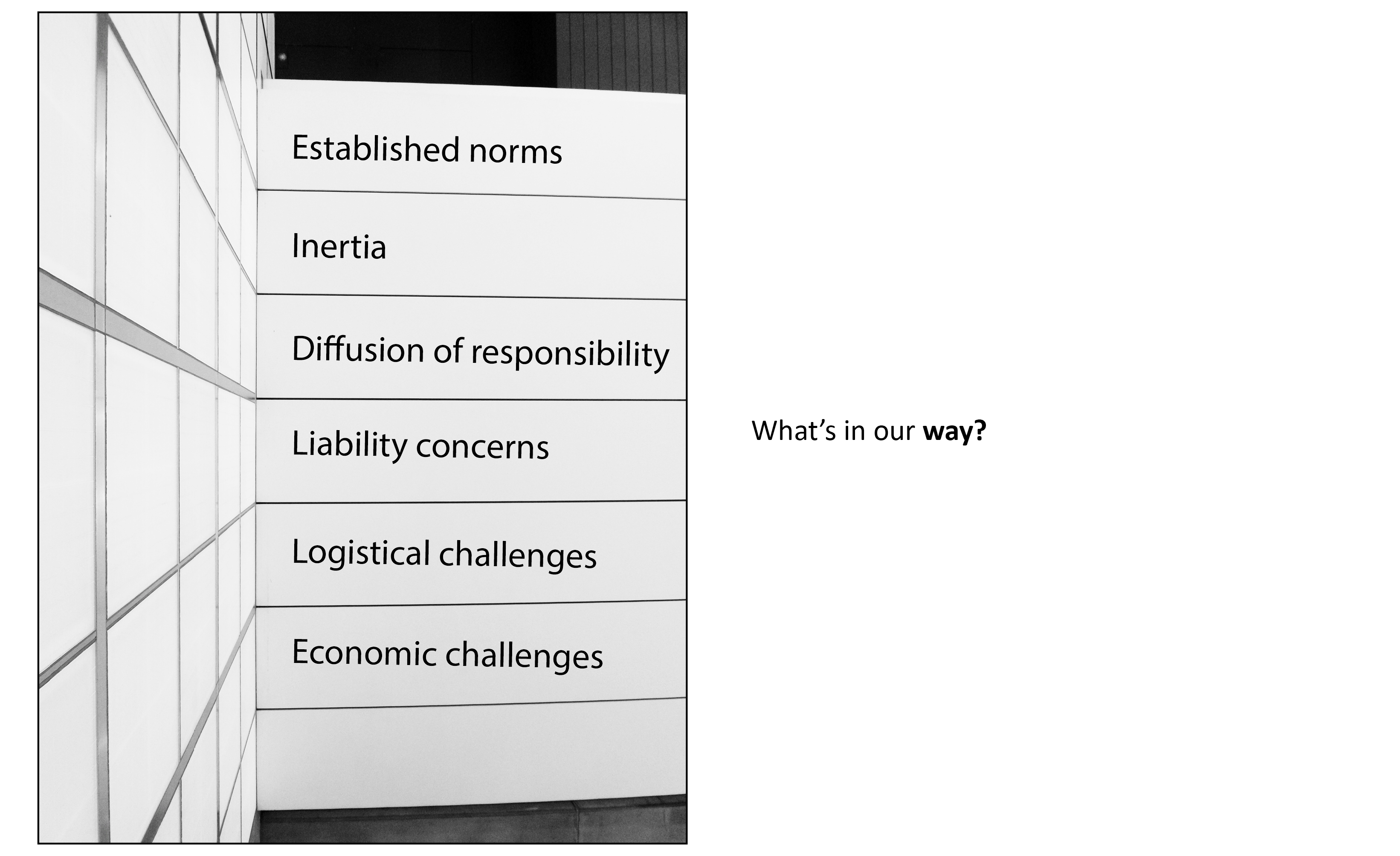
To foster acceptance, platforms like NeurIPS could adopt a registered reports format, pre-accepting dataset proposals to address financial uncertainties associated with ethical practices. Moreover, forming data consortia could help overcome operational hurdles (e.g., the implementation and maintenance of consent management systems) faced by smaller organizations and academic research groups through resource and knowledge pooling.
Extending our recommendations to the curation of "democratizing" foundation model-sized training datasets35, 36, 37, 38 poses an economic challenge. However, it is worth considering that "solutions which resist scale thinking are necessary to undo the social structures which lie at the heart of social inequality"39. Large-scale, nonconsensual datasets driven by scale thinking have included harmful and distressing content, including rape(missing reference), racist stereotypes40, vulnerable persons41, and derogatory taxonomies42, 43, 44, 45. Such content may further generate legal concerns46. We contend that these issues can be mitigated through the implementation of our recommendations.
Balancing resources between model development and data curation is value-laden, shaped by "social, political, and ethical values"47. While organizations readily invest significantly in model training48, 49, compensation for data contributors often appears neglected50, 51, disregarding that "most data represent or impact people"52. Remedial actions could be envisioned to bridge the gap between models developed with ethically curated data and those benefiting from expansive, nonconsensually crawled data. Reallocating research funds away from dominant data-hungry methods47 would help to strike a balance between technological advancement and ethical imperatives.
However, the granularity and comprehensiveness of our diversity recommendations could be adapted beyond evaluation contexts, particularly when employing "fairness without demographics"53, 54, 55, 56 training approaches, reducing financial costs. Nevertheless, the applicability of any proposed recommendation is intrinsically linked to the specific context57. Decisions should be guided by the social framework of a given application to ensure ethical and equitable data curation.
Just as the concepts of identity evolve, our recommendations must also evolve to ensure their ongoing relevance and sensitivity. Thus, we encourage dataset creators to tailor our recommendations to their context, fostering further discussions on responsible data curation.
References
- D. Raji, E. Denton, E. M. Bender, A. Hanna, and A. Paullada, “AI and the Everything in the Whole Wide World Benchmark,” in Advances in Neural Information Processing Systems Track on Datasets and Benchmarks (NeurIPS D&B), 2021.
- L. P. Nijhawan et al., “Informed consent: Issues and challenges,” Journal of advanced pharmaceutical technology & research, vol. 4, no. 3, p. 134, 2013.
- National Commission for the Proptection of Human Subjects of Biomedicaland Behavioral Research, Bethesda, Md, The Belmont report: Ethical principles and guidelines for the protection of human subjects of research. Superintendent of Documents, 1978.
- N. Code, “The Nuremberg Code,” Trials of war criminals before the Nuremberg military tribunals under control council law, vol. 10, no. 2, pp. 181–182, 1949.
- E. Politou, E. Alepis, and C. Patsakis, “Forgetting personal data and revoking consent under the GDPR: Challenges and proposed solutions,” Journal of cybersecurity, vol. 4, no. 1, p. tyy001, 2018.
- L. Edwards, “Privacy, security and data protection in smart cities: A critical EU law perspective,” Eur. Data Prot. L. Rev., vol. 2, p. 28, 2016.
- K. Karkkainen and J. Joo, “Fairface: Face attribute dataset for balanced race, gender, and age for bias measurement and mitigation,” in IEEE Winter Conference on Applications of Computer Vision (WACV), 2021, pp. 1548–1558.
- T.-Y. Lin et al., “Microsoft coco: Common objects in context,” in European Conference on Computer Vision (ECCV), 2014, pp. 740–755.
- J. Deng, W. Dong, R. Socher, L.-J. Li, K. Li, and L. Fei-Fei, “Imagenet: A large-scale hierarchical image database,” in IEEE/CVF Conference on Computer Vision and Pattern Recognition (CVPR), 2009, pp. 248–255.
- T. Karras, S. Laine, and T. Aila, “A style-based generator architecture for generative adversarial networks,” in IEEE/CVF Conference on Computer Vision and Pattern Recognition (CVPR), 2019, pp. 4401–4410.
- W. Kay et al., “The kinetics human action video dataset,” arXiv preprint arXiv:1705.06950, 2017.
- M. Andriluka, L. Pishchulin, P. Gehler, and B. Schiele, “2d human pose estimation: New benchmark and state of the art analysis,” in IEEE/CVF Conference on Computer Vision and Pattern Recognition (CVPR), 2014, pp. 3686–3693.
- M. Cordts et al., “The cityscapes dataset for semantic urban scene understanding,” in IEEE/CVF Conference on Computer Vision and Pattern Recognition (CVPR), 2016, pp. 3213–3223.
- S. Sarkar, P. J. Phillips, Z. Liu, I. R. Vega, P. Grother, and K. W. Bowyer, “The humanid gait challenge problem: Data sets, performance, and analysis,” IEEE Transactions on Pattern Analysis and Machine Intelligence, vol. 27, no. 2, pp. 162–177, 2005.
- Y. Xiong, K. Zhu, D. Lin, and X. Tang, “Recognize complex events from static images by fusing deep channels,” in IEEE/CVF Conference on Computer Vision and Pattern Recognition (CVPR), 2015, pp. 1600–1609.
- S. Yang, P. Luo, C.-C. Loy, and X. Tang, “Wider face: A face detection benchmark,” in IEEE/CVF Conference on Computer Vision and Pattern Recognition (CVPR), 2016, pp. 5525–5533.
- G. B. Huang, M. Mattar, T. Berg, and E. Learned-Miller, “Labeled faces in the wild: A database forstudying face recognition in unconstrained environments,” in Workshop on faces in’Real-Life’Images: detection, alignment, and recognition, 2008.
- O. Jesorsky, K. J. Kirchberg, and R. W. Frischholz, “Robust face detection using the hausdorff distance,” in Audio-and Video-Based Biometric Person Authentication: Third International Conference, AVBPA 2001 Halmstad, Sweden, June 6–8, 2001 Proceedings 3, 2001, pp. 90–95.
- A. Geiger, P. Lenz, and R. Urtasun, “Are we ready for autonomous driving? the kitti vision benchmark suite,” in IEEE/CVF Conference on Computer Vision and Pattern Recognition (CVPR), 2012, pp. 3354–3361.
- N. Dalal and B. Triggs, “Histograms of oriented gradients for human detection,” in IEEE/CVF Conference on Computer Vision and Pattern Recognition (CVPR), 2005, vol. 1, pp. 886–893.
- A. Angelova, Y. Abu-Mostafam, and P. Perona, “Pruning training sets for learning of object categories,” in IEEE/CVF Conference on Computer Vision and Pattern Recognition (CVPR), 2005, pp. 494–501.
- M. Everingham, L. Van Gool, C. K. I. Williams, J. Winn, and A. Zisserman, “The pascal visual object classes (voc) challenge,” International journal of computer vision, vol. 88, no. 2, pp. 303–338, 2010.
- Z. Liu, P. Luo, X. Wang, and X. Tang, “Deep learning face attributes in the wild,” in IEEE International Conference on Computer Vision (ICCV), 2015, pp. 3730–3738.
- J. Buolamwini and T. Gebru, “Gender shades: Intersectional accuracy disparities in commercial gender classification,” in ACM Conference on Fairness, Accountability, and Transparency (FAccT), 2018, pp. 77–91.
- L. A. Hendricks, K. Burns, K. Saenko, T. Darrell, and A. Rohrbach, “Women also snowboard: Overcoming bias in captioning models,” in European Conference on Computer Vision (ECCV), 2018, pp. 771–787.
- M. Mitchell et al., “Model cards for model reporting,” in ACM Conference on Fairness, Accountability, and Transparency (FAccT), 2019, pp. 220–229.
- M. K. Scheuerman, A. Hanna, and E. Denton, “Do datasets have politics? Disciplinary values in computer vision dataset development,” Proceedings of the ACM on Human-Computer Interaction, vol. 5, no. CSCW2, pp. 1–37, 2021.
- M. K. Andrus, E. Spitzer, and A. Xiang, “Working to Address Algorithmic Bias? Don’t Overlook the Role of Demographic Data,” Partnership on AI, 2020.
- M. K. Andrus, E. Spitzer, J. Brown, and A. Xiang, “What We Can’t Measure, We Can’t Understand: Challenges to Demographic Data Procurement in the Pursuit of Fairness,” in ACM Conference on Fairness, Accountability, and Transparency (FAccT), 2021, pp. 249–260.
- J. T. A. Andrews, P. Joniak, and A. Xiang, “A View From Somewhere: Human-Centric Face Representations,” in International Conference on Learning Representations (ICLR), 2023.
- D. Zhao, J. T. A. Andrews, and A. Xiang, “Men Also Do Laundry: Multi-Attribute Bias Amplification,” in International Conference on Machine Learning (ICML), 2023.
- J. Metcalf and K. Crawford, “Where are human subjects in big data research? The emerging ethics divide,” Big Data & Society, vol. 3, no. 1, p. 2053951716650211, 2016.
- A. Birhane, “Automating ambiguity: Challenges and pitfalls of artificial intelligence,” arXiv preprint arXiv:2206.04179, 2022.
- S. Hooker, “Moving beyond ‘algorithmic bias is a data problem,’” Patterns, vol. 2, no. 4, p. 100241, 2021.
- P. Goyal et al., “Vision models are more robust and fair when pretrained on uncurated images without supervision,” arXiv preprint arXiv:2202.08360, 2022.
- C. Schuhmann et al., “Laion-400m: Open dataset of clip-filtered 400 million image-text pairs,” arXiv preprint arXiv:2111.02114, 2021.
- C. Schuhmann et al., “Laion-5b: An open large-scale dataset for training next generation image-text models,” Advances in Neural Information Processing Systems (NeurIPS), vol. 35, pp. 25278–25294, 2022.
- S. Y. Gadre et al., “DataComp: In search of the next generation of multimodal datasets,” arXiv preprint arXiv:2304.14108, 2023.
- A. Hanna and T. M. Park, “Against scale: Provocations and resistances to scale thinking,” arXiv preprint arXiv:2010.08850, 2020.
- A. Hanna, E. Denton, R. Amironesei, A. Smart, and H. Nicole, “Lines of Sight,” Logic(s). https://logicmag.io/commons/lines-of-sight/, 2020.
- H. Han, A. K. Jain, F. Wang, S. Shan, and X. Chen, “Heterogeneous face attribute estimation: A deep multi-task learning approach,” IEEE Transactions on Pattern Analysis and Machine Intelligence, vol. 40, no. 11, pp. 2597–2609, 2017.
- B. Koch, E. Denton, A. Hanna, and J. G. Foster, “Reduced, Reused and Recycled: The Life of a Dataset in Machine Learning Research,” in Advances in Neural Information Processing Systems Datasets and Benchmarks Track (NeurIPS D&B), 2021.
- A. Birhane and V. U. Prabhu, “Large image datasets: A pyrrhic win for computer vision?,” in IEEE Winter Conference on Applications of Computer Vision (WACV), 2021, pp. 1536–1546.
- M. Hanley, A. Khandelwal, H. Averbuch-Elor, N. Snavely, and H. Nissenbaum, “An Ethical Highlighter for People-Centric Dataset Creation,” Advances in Neural Information Processing Systems Workshop (NeurIPSW), 2020.
- K. Crawford and T. Paglen, “Excavating AI: The politics of images in machine learning training sets,” Ai & Society, vol. 36, no. 4, pp. 1105–1116, 2021.
- “OpenAI and Microsoft Sued for $3 Billion Over Alleged ChatGPT ’Privacy Violations’ — vice.com.” https://www.vice.com/en/article/wxjxgx/openai-and-microsoft-sued-for-dollar3-billion-over-alleged-chatgpt-privacy-violations.
- B. Blili-Hamelin and L. Hancox-Li, “Making Intelligence: Ethical Values in IQ and ML Benchmarks,” in ACM Conference on Fairness, Accountability, and Transparency (FAccT), 2023, pp. 271–284.
- E. Mostaque (Stability AI), “We actually used 256 A100s for this per the model card, 150k hours in total so at market price $600k,” Twitter. https://twitter.com/emostaque/status/1563870674111832066, 28-Aug-2022.
- J. Vincent, “‘An engine for the imagination’: the rise of AI image generators. An interview with Midjourney founder David Holz.” The Verge, 03-Aug-2022.
- J. Vincent, “Getty Images is suing the creators of AI art tool stable diffusion for scraping its content,” The Verge. https://www.theverge .com/2023/1/17/23558516/ai-art-copyright-stable-diffusion-getty-images-lawsuit, Jan-2023.
- J. Vincent, “AI art tools stable diffusion and Midjourney targeted with copyright lawsuit,” The Verge. https://www.theverge.com/2023/1/16/23557098/generative-ai-art-copyright-legal-lawsuit-stable-diffusion-midjourney-deviantart, Jan-2023.
- M. Zook et al., “Ten simple rules for responsible big data research,” PLoS computational biology, vol. 13, no. 3. Public Library of Science San Francisco, CA USA, p. e1005399, 2017.
- N. L. Martinez, M. A. Bertran, A. Papadaki, M. Rodrigues, and G. Sapiro, “Blind pareto fairness and subgroup robustness,” in International Conference on Machine Learning, 2021, pp. 7492–7501.
- P. Lahoti et al., “Fairness without demographics through adversarially reweighted learning,” Advances in Neural Information Processing Systems (NeurIPS), vol. 33, pp. 728–740, 2020.
- T. Hashimoto, M. Srivastava, H. Namkoong, and P. Liang, “Fairness without demographics in repeated loss minimization,” in International Conference on Machine Learning, 2018, pp. 1929–1938.
- J. Chai, T. Jang, and X. Wang, “Fairness without demographics through knowledge distillation,” Advances in Neural Information Processing Systems (NeurIPS), vol. 35, pp. 19152–19164, 2022.
- O. Papakyriakopoulos et al., “Augmented Datasheets for Speech Datasets and Ethical Decision-Making,” in ACM Conference on Fairness, Accountability, and Transparency (FAccT), 2023, pp. 881–904.
Acknowledgments
This work was funded by Sony Research Inc.